Who Calls the Shots? Rethinking Few-Shot Learning for Audio
Wang, Y., Bryan, N., Salamon, J., Cartwright, M., Bello, J.P. Who calls the shots? Rethinking few-shot learning for audio. In Proceedings of the IEEE Workshop on Applications of Signal Processing to Audio and Acoustics (WASPAA), 2021. Special Best Paper Award
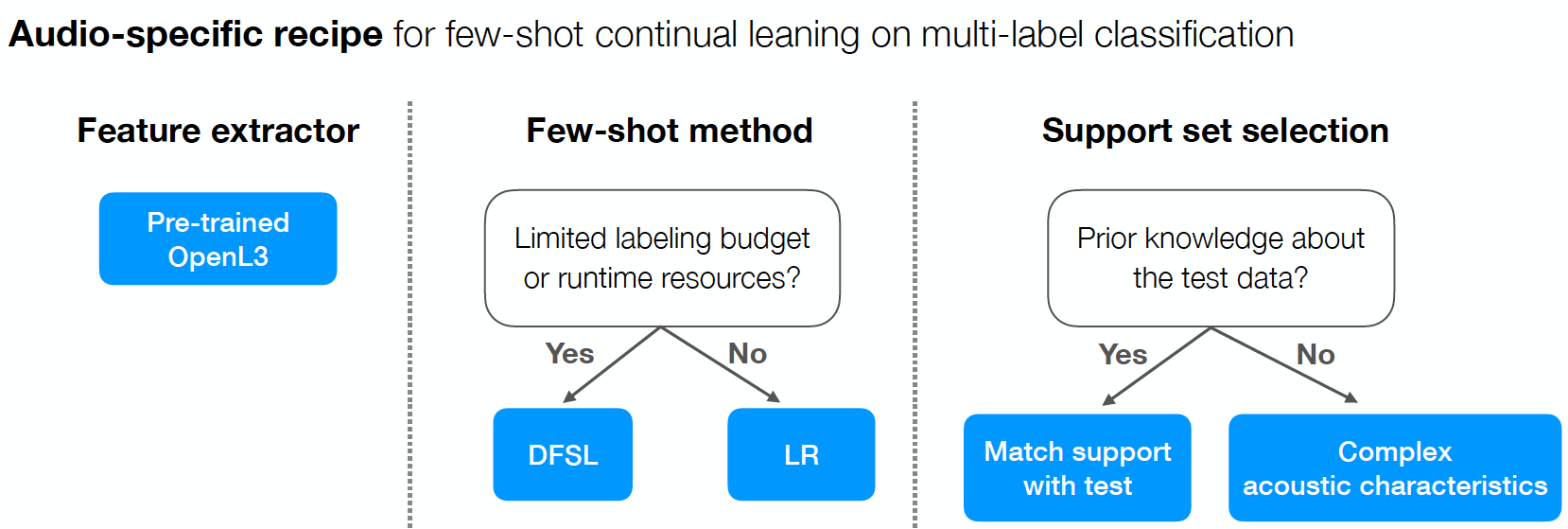
Abstract
While the field has seen notable advances in recent years, they have often focused on multi-class image classification. Audio, in contrast, is often multi-label due to overlapping sounds, resulting in unique properties such as polyphony and signal-to-noise ratios (SNR). This leads to unanswered questions concerning the impact such audio properties may have on few-shot learning system design, performance, and human-computer interaction, as it is typically up to the user to collect and provide inference-time support set examples. We address these questions through a series of experiments designed to elucidate the answers to these questions. We introduce two novel datasets, FSD-MIX-CLIPS and FSD-MIX-SED, whose programmatic generation allows us to explore these questions systematically. Our experiments lead to audio-specific insights on few-shot learning, some of which are at odds with recent findings in the image domain: there is no best one-size-fits-all model, method, and support set selection criterion. Rather, it depends on the expected application scenario.
Resources
- Code: Code repository
- Data: FSD-MIX-CLIPS