Specialized Embedding Approximation for Edge Intelligence: A Case Study in Urban Sound Classification
Srivastava, S., Roy, D., Cartwright, M., Bello, J.P., Arora, A. Specialized Embedding Approximation for Edge Intelligence: A Case Study in Urban Sound Classification. In Proceedings of the IEEE International Conference on Acoustics, Speech and Signal Processing (ICASSP), 2021.
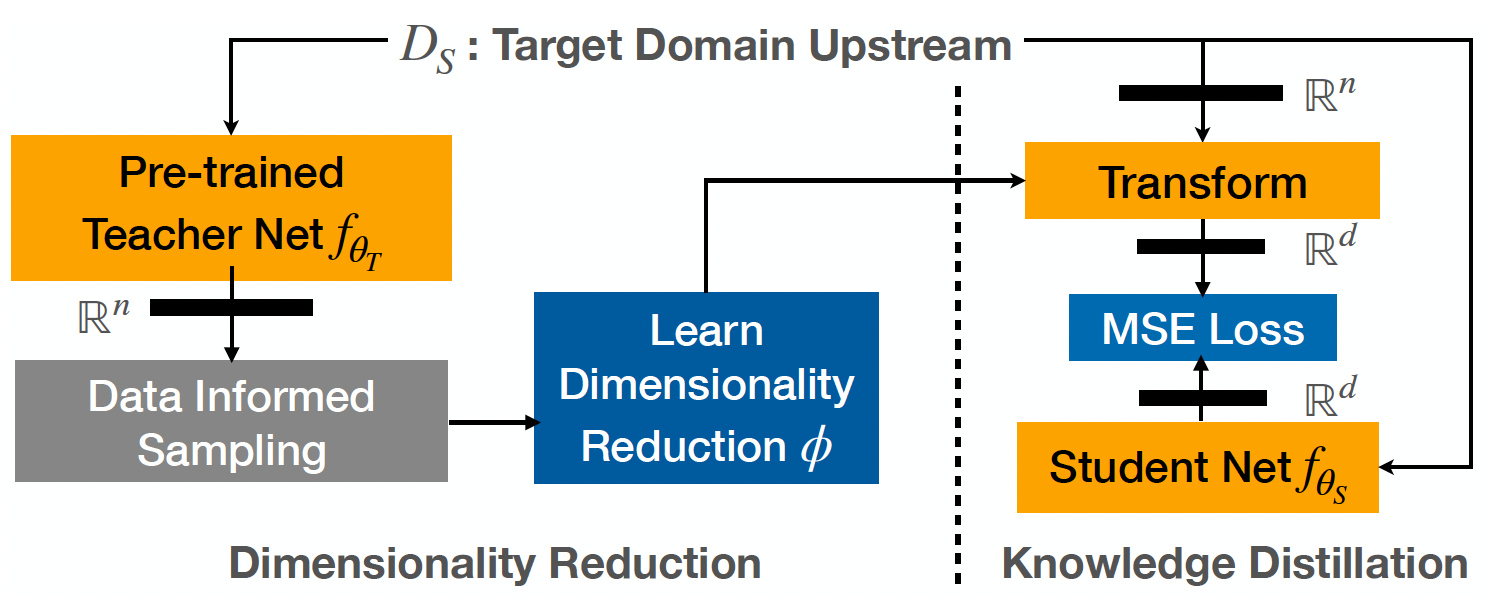
Abstract
Embedding models that encode semantic information into low-dimensional vector representations are useful in various machine learning tasks with limited training data. However, these models are typically too large to support inference in small edge devices, which motivates training of smaller yet comparably predictive student embedding models through knowledge distillation (KD). While knowledge distillation traditionally uses the teacher’s original training dataset to train the student, we hypothesize that using a dataset similar to the student’s target domain allows for better compression and training efficiency for the said domain, at the cost of reduced generality across other (non-pertinent) domains. Hence, we introduce Specialized Embedding Approximation (SEA) to train a student featurizer to approximate the teacher's embedding manifold for a given target domain. We demonstrate the feasibility of SEA in the context of acoustic event classification for urban noise monitoring and show that leveraging a dataset related to this target domain not only improves the baseline performance of the original embedding model but also yields competitive students with > 1 order of magnitude lesser storage and activation memory. We further investigate the impact of using random and informed sampling techniques for dimensionality reduction in SEA.
Resources
- Code: EdgeL3 python package